- AI Street
- Posts
- Outsmarting the market with AI: An Interview with Dr. Lopez-Lira on his new book
Outsmarting the market with AI: An Interview with Dr. Lopez-Lira on his new book
Q & A

AI Street interviewed Dr. Alejandro Lopez-Lira, a finance professor at the University of Florida and author of “The Predictive Edge: Outsmart the Market using Generative AI and ChatGPT in Financial Forecasting” out today.
The book explains how to use artificial intelligence and large language models (LLMs) to find investment ideas, improve trading strategies and increase efficiency.
Lopez-Lira has been at the forefront of AI and finance, publishing a research paper last year on ChatGPT’s ability to predict stock returns based on news headlines. Lopez-Lira and his co-author, Yuehua Tang, asked ChatGPT to decide how a headline would affect a company’s stock price with a short prompt:
Forget all your previous instructions. Pretend you are a financial expert. You are a financial expert with stock recommendation experience. Answer “YES” if good news, “NO” if bad news, or “UNKNOWN” if uncertain in the first line. Then elaborate with one short and concise sentence on the next line. Is this headline good or bad for the stock price of [X] company?
ChatGPT produced statistically significant results that outperformed traditional sentiment models despite it not having any explicit financial training.
The study, which has been downloaded more than 60,000 times, landed Lopez-Lira his first book deal and garnered interest from multiple news outlets, major hedge funds, and the U.S. Securities and Exchange Commission.
This interview has been edited for clarity and length.
AI Street: How did your book come about?
Everybody was interested in the research paper. I got calls from journalists, hedge funds. An editorial contact at Wiley said `Hey, you want to write a book about it?’
So, I did. And in the book, it runs from beginner to more advanced topics. You will learn the basics of AI and the basics of finance from risk management to summarization to more advanced tasks like having agents based on LLMs trading by themselves.
What can you currently do with this technology?
You can use LLMs to assess economic news like macroeconomic indicators and combine that to the specific program that's executing the software.
I created a markets’ website where all the content is generated by large language models. It generates a daily report with macroeconomic environment news, headlines on companies for every S&P 500 stock. I built this with my dad, who’s a software engineer and it took us about six hours or something.
This website is a kind of showcase of what you can already do with existing tools. You can take a bunch of unstructured data: news, conference calls, whatever you want and produce nice reports.
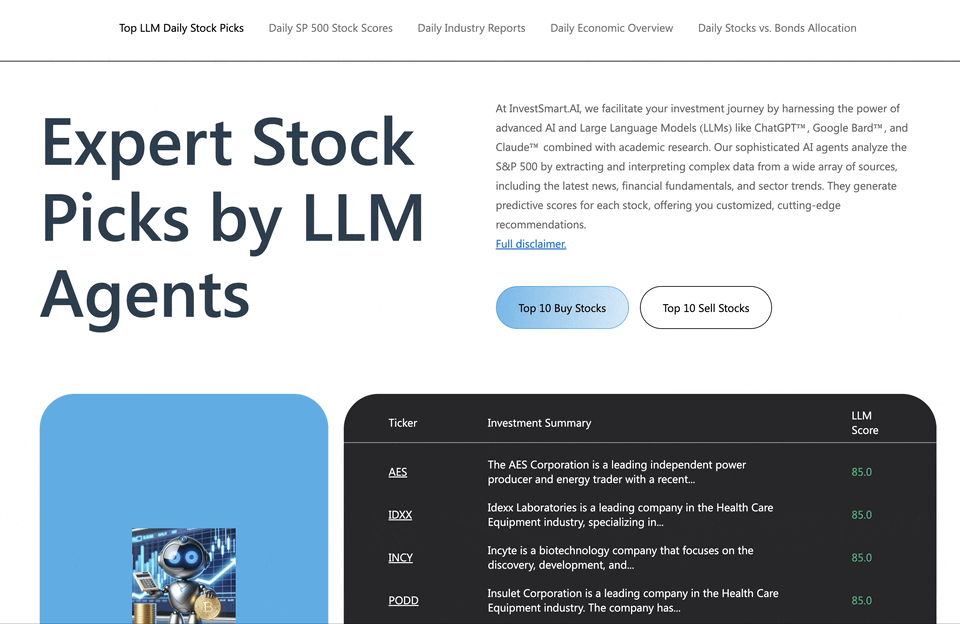
How do you minimize hallucinations?
Once large language models’ answers become very long, they start to degrade quickly. You need to keep the output short. Just breaking tasks into very small parts, and then combining them, goes a long way.
It’s always a very, very good idea to ask the LLM: Does this seem correct to you? Like, are there any big red flags? And then you can flag that automatically and you can have a Python script that does that. If you're doing something that's super, super sensitive, then I would really just make sure simple tasks are checked, and then combined and then checked again. That tends to work relatively well.
What are some other use cases?
It can streamline a lot of work. Before you had to have analysts reading the news all day and then writing a report. Now, it takes like 30 seconds to do it with a large language model.
You can also use AI to explain a portfolio’s performance. Before, to create a portfolio report, it was a lot of work since you had to have someone tracking these stocks and explain what happened. Now you'll just pass it on to a large language model, ask it to format it into a nice report. So bam, it’s personalized finance for everyone. This is also one of the speculative use cases that I discussed a little bit in the book.
What LLMs do you use?
I use a good mix. I like Claude by Anthropic a lot because the web version seems to understand a document globally. Other large language models, when you upload a big document, it seems they're just getting small chunks of the text, so they don't have a global structure.
Claude can criticize academic research extremely well, for example, at an insane level. I spoke with some professors and they were so impressed, because this is almost at the academic level of criticizing a paper and it's way, way, way above the average human that has no expertise in finance.
And then with ChatGPT what I love is that you can quickly prototype something because it can code. If I have an idea, I could just ask it to simulate it in Python, and then build a small prototype, right away.
What’s underhyped and overhyped in AI?
I think underhyped is anything that can be broken into small tasks. We can already do that with existing models. Anything that an intern can do without a lot of training, you can do right now with the current models.
Overhyped? It’s probably the trading applications. Trading is a zero sum game. So it's all about execution and looking at different signals, rather than just analyzing the information. It's not going to change trading quite so deeply.
How does AI impact investors?
It used to be the case that the most advanced technology tools to forecast returns were only available to very large companies. Now, the best tools are available to basically anyone. AI is going to democratize access to these technologies.
How is AI going to affect the workforce?
A lot of people are worried that this is going to take their jobs. And the answer is that jobs are made of tasks. And a lot of tasks are amenable for large language models, but most tasks are not. Open-ended tasks are still way above the model capabilities.
Reality is very complex, and language only captures a little bit of it. Building a house from scratch, it's something that I don't think we're going to be solving in three years.
What will the world of AI and finance look like in five years?
I expect that local models are going to become easier to host and smarter. You’d take an open-source model, fine tune it without it ever touching the internet. A lot of tasks that are sensitive will be done with AI in five years for sure. Whereas now there may be some concern that “hey, I don't know if I can send my client’s information” [to an AI provider]. In five years, you will not need to send anything to anyone.
Anything that involves personalized finance, I think will grow a lot because before it was too costly. Now it's super easy to write explanations to clients about what happened. Also, it’ll have a big impact on thematic investing. It's trivially easy to get a list of certain companies for a certain theme. And I'll have an ETF by tomorrow morning.
Thanks for reading! Who should we interview next? Reach out to [email protected].

Reply